AI Agents: Transforming Data Analytics Through Agentic AI


AI agents—intelligent software that perceives environments, makes decisions, and takes actions to achieve specific goals—are fundamentally reshaping how people interact with data and systems. AI agents are popping up everywhere: agents for sales, customer support, legal—heck, there’s even one for fortune-telling! With the market for AI agents expected to grow from $5.1 billion today to a $47.1 billion market by 2030, there is a meteoric rise ahead. This post will explore how AI agents are taking enterprise data analysis to a new level, and what to expect in this new agentic analytics era.
What are AI agents? And what is agentic analytics?
AI agents are intelligent, generative AI (GenAI)-powered software leveraging planning, short and long-term memory, tools, and learnings to autonomously decide what to do and when to do it. Think ChatGPT for complex actions and goals—complex multistep processes to achieve goals—and not just conversations.
Agentic analytics is the application of AI agents for data analysis and insight generation. In the context of data analytics, AI agents can carry out tasks like data exploration, pattern recognition, predictive modeling, and decision- and action-making—often at a scale and speed beyond human capabilities. The key part, though, is they can operate autonomously, creating a comprehensive decision workflow or sophisticated analysis that might otherwise be conducted by a management consultant or take analyst teams days to do.
How are AI agents different from chatbots and LLM copilots?
AI agents are distinct from chatbots and LLM-powered copilots in several ways:

AI agents—more adaptive and proactive—can plan and tackle complex, multi-step processes to achieve goals, rather than being pre-programmed or directed by humans at each step. They operate in complex environments and leverage a multitude of skills and tools. The key difference is the level of autonomy or agency they possess.
How is agentic analytics different from traditional and AI-assisted analytics?
In similar ways to how AI agents are different from chatbots and copilots, agentic analytics is different from traditional and AI-assisted approaches to analytics. Basically replace the terms in the above chart: “Chatbot” with “Traditional Analytics,” “Copilots” with “AI-Assisted Analytics,” and “AI Agents” with “Agentic Analytics.” In more detail, the key differences are:
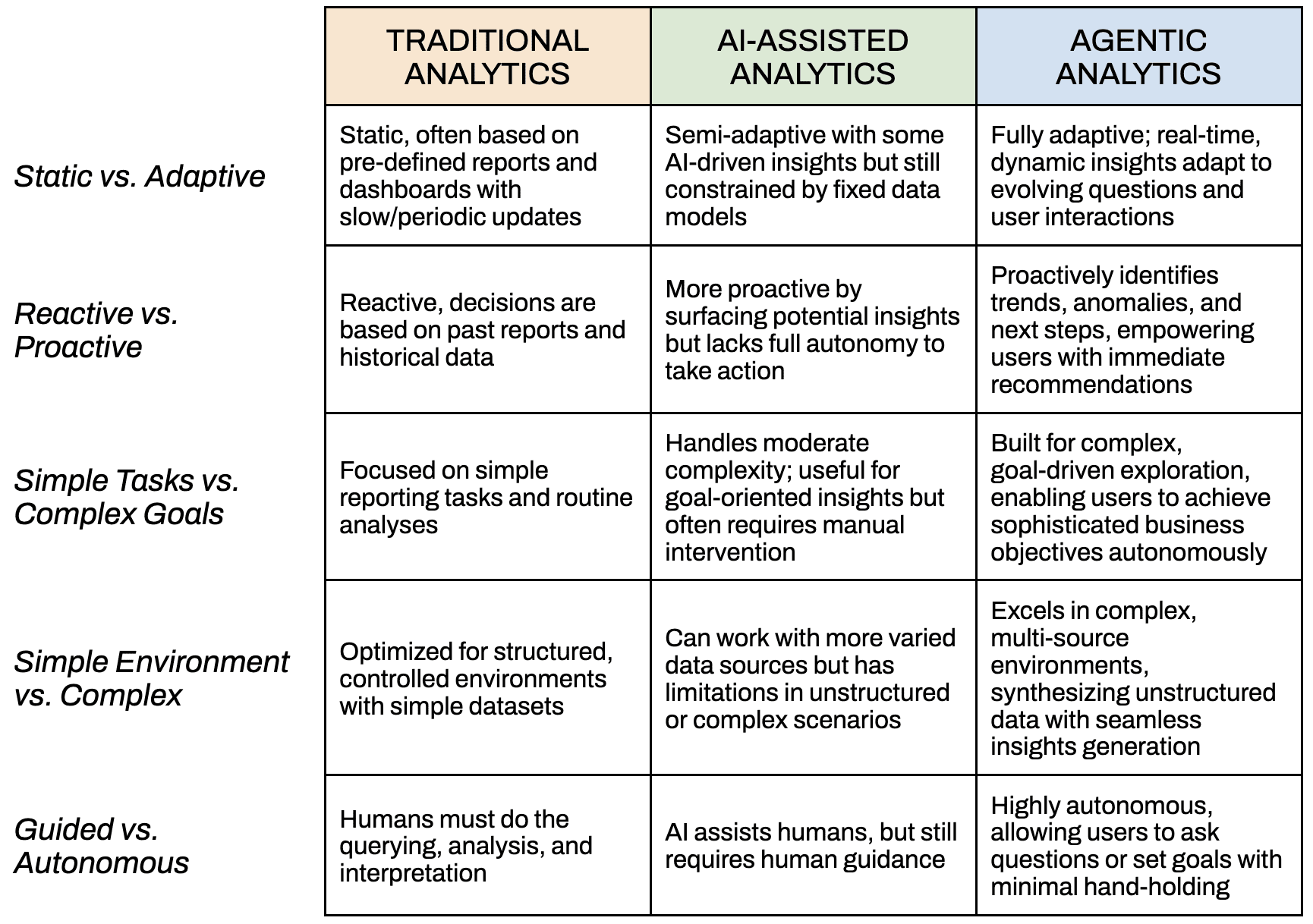
Agentic analytics, powered by generative AI (i.e., large language models), acts autonomously by setting its own goals, making decisions, and interacting with the world in a dynamic way, mimicking human-like decision-making by processing real-time data and learning from its experiences to achieve predefined objectives. What sets it apart is its autonomy, as it’s capable of operating independently, reacting to environmental changes, and handling complex tasks without constant human prompting.
Generative AI on its own, without agentic capabilities, is focused on creating new content based on the data it’s been trained on by a human. It doesn’t inherently “act” in the world or make decisions about goals—instead, its strength lies in its ability to generate outputs, like the ever prevalent chatbot that drafts text or the AI image generator that creates visual art.
A generative AI model like GPT processes input prompts from users and creates content in response, but it doesn’t make decisions on what tasks to pursue, or how to approach those tasks independently. Its functionality is narrower in the sense that it needs clear inputs to produce outputs. It doesn’t engage in the more complex, goal-driven behaviors that are typical of agentic AI.
What can agentic analytics do?
Agentic analytics takes the drudgery out of data exploration, pattern detection, advanced analysis, augmentation/automation, and personalization.
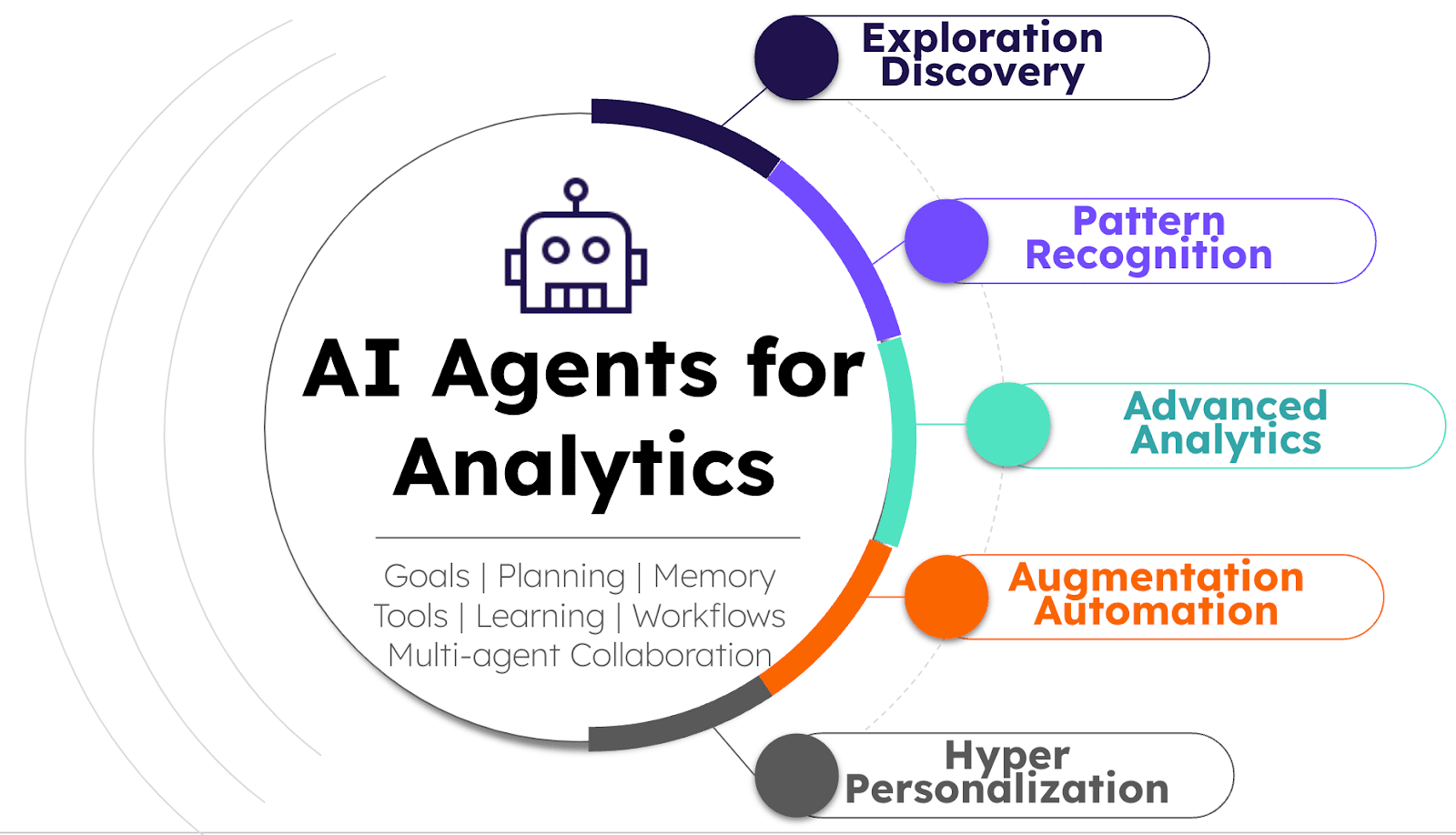
Breaking these down further, there are numerous analytics use cases for agents:

- ADVANCED DATA EXPLORATION AND DISCOVERY
- Data cleansing & enrichment. Agentic analytics autonomously identifies, cleans, and enriches raw datasets, recognizing and correcting inconsistencies, and appending relevant external data and interacting (and in some cases, adjusting) the semantic layer to ensure analysis-ready data with minimal user intervention.
- Conversational analytics and ad hoc analysis. Users can ask complex, nuanced questions in natural language, and AI analyst agents can accurately understand the question’s intent, route the query to the underlying data (or pull in the necessary data—see previous point) to provide immediate, insightful responses and visualizations and exploratory paths for in-depth, real-time analyses on the fly. For example, agents could tackle open-ended questions like, “How can my organization increase overall sales? How can we improve the market share of this particular drug?”
- Hypothesis generation/testing. Agentic analytics proactively generates analysis hypotheses based on patterns in the data and tests them (work that analysts and business users typically do), surfacing statistically significant findings and highlighting actionable insights without requiring manual setup.
- PATTERN RECOGNITION
- Automated insights generation. Agents continuously scan billions of datapoints across numerous disparate datasets to autonomously generate insights, uncovering emerging trends, patterns, and hidden relationships that drive data-driven decisions without prompting. Think of an AI agent as a tireless assistant who can sift through massive amounts of data, finding patterns and anomalies that even the sharpest human analyst might miss. AI agents are speeding up data discovery and uncovering extremely complex insights that would pretty much be a nightmare to find manually.
- Anomaly detection. AI agents can spot important changes or events as they happen and either take action right away or give decision-makers a heads-up with contextual explanations of potential impacts: e.g. “Hey, there’s a supply chain issue in the Northeast U.S. that may affect supply of a product in the coming months!”
- Root cause analysis. When anomalies or shifts occur, agentic analytics quickly traces data back to identify underlying causes, providing users with comprehensive, actionable explanations to inform corrective actions.
- ADVANCED ANALYTICS
- Real-time analytics. Agentic analytics delivers instant insights by continuously monitoring data streams, making informed decisions in real time as new information becomes available—or passing along this information to humans to take action rapidly.
- Predictive and prescriptive analytics. Leveraging historical data and machine learning, agentic analytics forecasts future trends and outcomes, equipping users with anticipatory insights to optimize strategies proactively: e.g., “Seeing what’s coming up ahead, let’s ramp up supply of that product now so we don’t have an issue down the line.”
- Prescriptive / what-if scenario planning. Agentic analytics enables users to simulate various scenarios and receive data-driven recommendations on optimal actions, supporting strategic decision-making by predicting real-world impact by considering multi-dimensional relationships—analyzing problems from multiple perspectives.
- AUTOMATED REPORT GENERATION AND DATA NARRATIVES
- Automated report generation. Autonomous agents automatically compile and customize reports, integrating the latest insights and visualizations tailored to specific user needs without requiring manual setup.
- Automated narratives/summaries. Agentic analytics generates natural language summaries and narratives, translating complex data findings into clear, actionable insights that are more digestible and accessible to end-users.
- Multistep complex analysis workflows. Agentic analytics autonomously orchestrates multi-step analytical processes, executing complex workflows that include data preparation, analysis, and result interpretation, all tailored to user-defined objectives.
- HYPER-PERSONALIZED ANALYTICS
- Adaptive user interfaces. AI agents can provide highly personalized analytics experiences tailored to each user’s preferences, showing them exactly what they need in the way they prefer to see it. For example, a CFO might prefer a tightly worded top-line briefing with links to recommended actions that impact financial and operational performance, whereas a marketing analyst might prefer to see real-time recommendations embedded in their preferred marketing tools for just-in-time actions.
- Continuous learning and model refinement. AI agents keep on getting smarter. They learn from every interaction with data and users, constantly improving their accuracy and adapting to changes, similar to how a human would.
What are the benefits of agentic AI for data analytics?
Agentic analytics is reshaping how businesses interact with data, unlocking greater efficiency, scalability, enhanced decision-making, and cost reductions:

Increased efficiency: AI agents analyze massive datasets faster than humans, providing real-time insights that allow businesses to respond quickly to market changes.
Scalability: Agentic AI can handle tasks at scale, making it ideal for organizations with large volumes of data or complex analytics needs.
Continuous learning: AI agents continuously learn from data and interactions, improving accuracy and outcomes over time.
Cost reduction: By automating routine analytics and decision-making tasks, businesses can cut costs and reallocate resources to more strategic areas.
Agentic analytics in the wild
Let’s explore how agentic analytics is transforming specific industries.
In the commercial pharmaceutical sector, for example, AI agents can dramatically expedite and improve commercial sales effectiveness. Rather than manually collecting, cleaning, enriching, benchmarking, segmenting, and recommending improved sales targeting approaches, AI agents can automatically spot sales effectiveness key drivers and take or recommend action to improve targeting strategies.
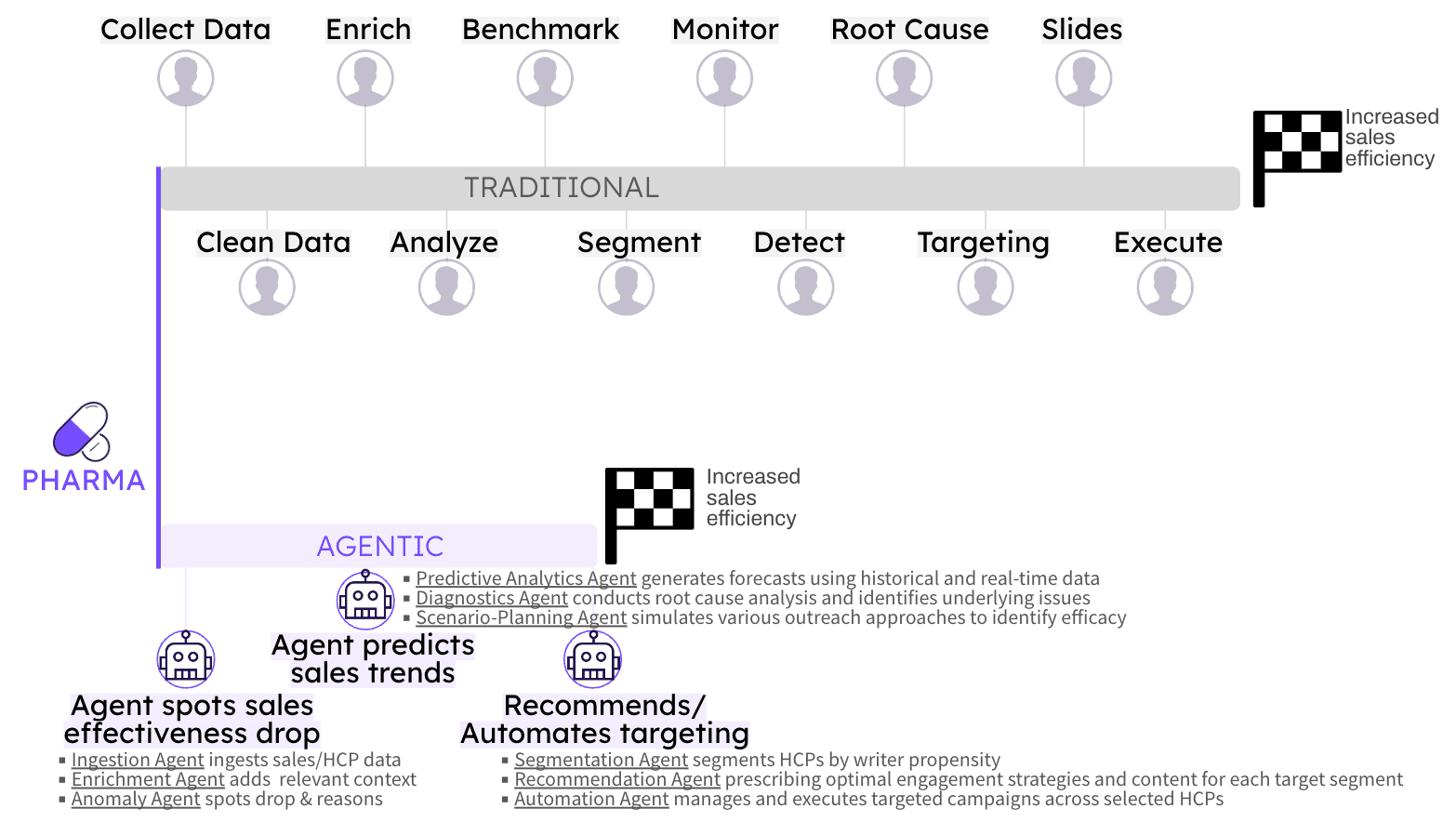
Now let’s look at the consumer packaged goods (CPG) industry, where AI agents are reshaping demand forecasting and supply chain optimization. They’re processing real-time sales data, social media trends, and even weather patterns to predict consumer behavior with unprecedented accuracy. In turn, this enables CPG firms to optimize inventory levels, reduce waste, and respond quickly to market changes.
Take Unilever, for instance, which is using AI agents to augment demand forecasting and supply chain optimization. The CPG giant is reportedly trying out a system that integrates real-time sales data, customer demand signals, and external factors like weather to optimize inventory levels and ensure product availability. The company partnered with Walmart in Mexico to pilot the model to significantly improve stock levels and reduce inventory waste. In the end, by leveraging agentic AI, Unilever not only optimizes inventory but also minimizes the need for manual intervention in forecasting.
In the high-tech industry, AI agents are enhancing everything from product design to customer support. They’re analyzing user behavior to inform feature development, predicting hardware failures before they occur, and providing personalized tech support through natural language interfaces.
Agentics analytics risks and mitigation strategies
It’s important to remember that current agentic systems rely heavily on LLMs, making them susceptible to the limitations of LLMs. Here are some key considerations and mitigation strategies for agentic analytics.
Hallucinations: Agentic system hallucinations could have negative impacts because it’s not just words but actions that go awry in a semi-automated or fully automated fashion.
🛠️ MITIGATION:
- Leverage a semantic layer for data consistency. Implementing a semantic layer for enterprise-grade AI agents ensures the AI references a unified source of truth, standardizing terminology and metrics across data sources to reduce the likelihood of hallucinated or inconsistent outputs.
- Apply reinforcement learning (RL) with human feedback. Incorporate RL models trained with human feedback to reinforce accurate outputs over time. Regular feedback cycles from experts help refine the AI’s responses, minimizing the chances of hallucination through continuous learning.
- Use retrieval-augmented generation (RAG) for fact-driven responses. By combining a retrieval mechanism with generation, RAG enables the AI to pull relevant, up-to-date information directly from verified databases, limiting hallucinations by grounding responses in factual data.
- Enforce enterprise-grade security for data integrity. Robust security protocols, including data access controls and secure API management, safeguard the data feeding the AI, maintaining high data quality and consistency. This reduces risks associated with using unverified or altered data that might lead to hallucinations.
Structured Data LLM Errors: Processing structured data with LLMs (necessary for analytics) faces potential errors at various stages, including:
- Converting text to SQL reliably
- Interpreting complex schemas
- Performing different types of analysis
🛠️ MITIGATION:
Leverage a semantic layer to streamline schema complexity, making it easier for the LLM to generate reliable SQL queries. Fine-tune models on specific schema data to improve their interpretation of unique structures, while RAG dynamically integrates schema references to enhance real-time accuracy in complex queries. Additionally, reinforcement learning enables models to learn from query outcomes, optimizing reliability over time.
Disengage after one bad experience: Business users have a very low tolerance for failure when it comes to semiautonomous and augmented systems. A single negative experience can easily deter users from future engagement.
🛠️ MITIGATION:
- Implement immediate feedback mechanisms. Provide users with real-time feedback options to report issues or suggest improvements directly within the system. This shows responsiveness to their concerns and enables quick adjustments that can restore confidence.
- Offer guided onboarding and support. Use interactive onboarding and continuous support resources to help users navigate the system confidently, reducing the likelihood of frustration. Reinforce successful interactions to build trust gradually.
- Showcase continuous learning and improvement. Highlight enhancements based on user feedback, demonstrating that the system evolves to meet their needs. Communicating updates helps rebuild trust and encourages users to re-engage, knowing their experience matters and drives tangible improvements.
The future of agentic AI in analytics: What’s next?
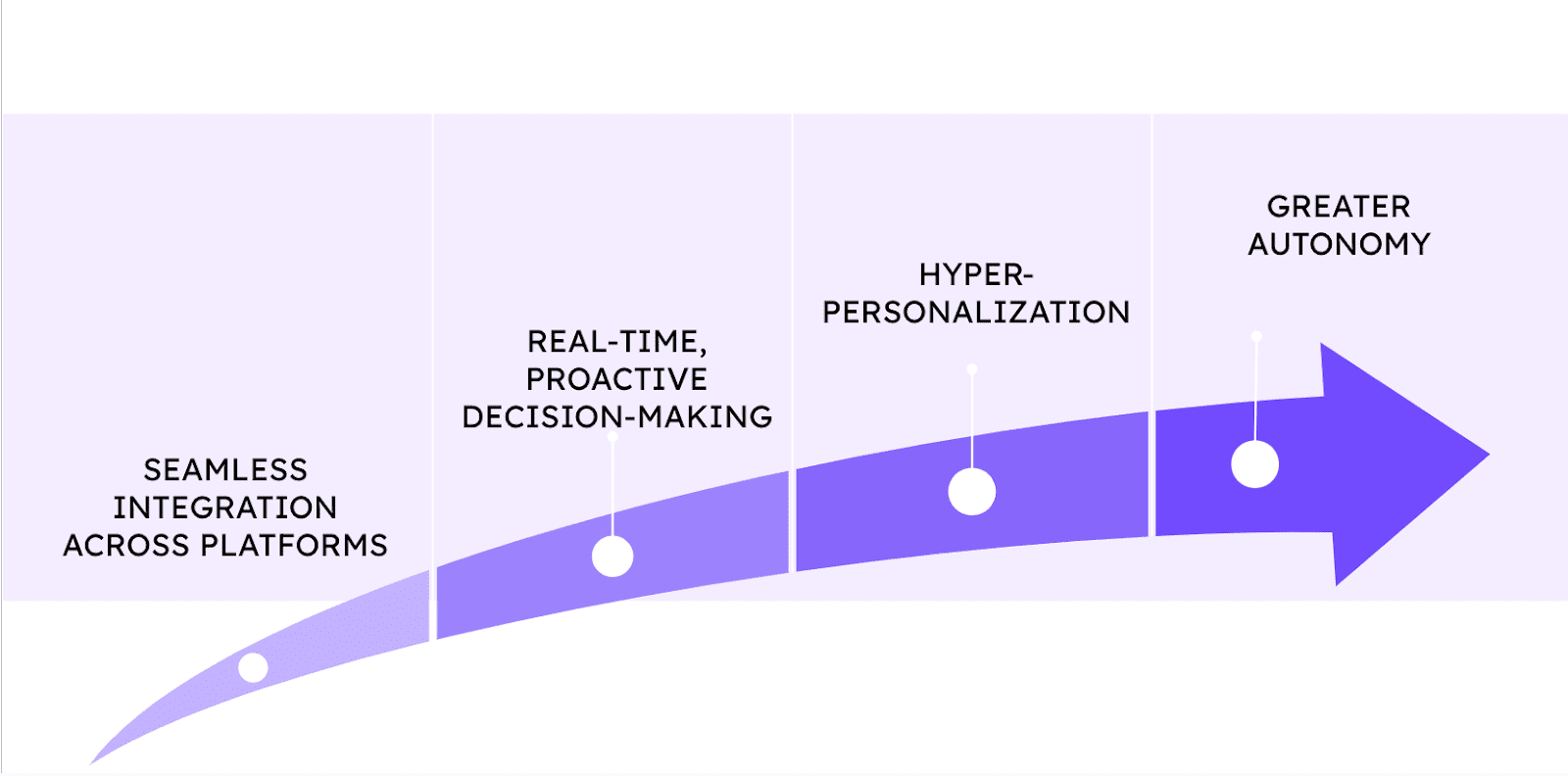
So, where is all this headed?
The future of agentic AI lies in its ability to seamlessly integrate reasoning, decision-making, and action across even more complex datasets and industries. Here’s what we can expect to see:
- Seamless integration: AI agents will interact across multiple platforms and datasets, providing a more unified view of the business. In the future, AI agent mesh or agent marketplaces could emerge, where best-in-class or pre-vetted agents could quickly plug-and-play, and more analytics and decisions could be made in an agent-to-agent manner (vs. good-old-fashioned human-based decisions.
- Real-time, proactive decision-making: AI agents will anticipate trends and make recommendations before problems arise.
- Hyper-personalized analytics: AI agents will provide highly tailored recommendations, analyzing every customer interaction in real time and autonomously adjusting strategies to maximize value.
- Even greater autonomy: AI agents will be able to handle more sophisticated tasks, breaking them down into actionable steps with minimal human intervention.
As we stand at the intersection of artificial intelligence and data analytics, the emergence of AI agents marks a paradigm shift in how we perceive and interact with data. This transformation goes way beyond technological advancement—it more so represents a fundamental reimagining of the relationship between human intelligence and machine capability.
But remember: The future of agentic analytics isn’t really just about smarter algorithms or more efficient data processing. It’s about fostering a symbiotic relationship between human creativity and machine intelligence (think: “human in the loop”). The most successful organizations will be those that can seamlessly continue to blend human intuition with AI-driven insights, creating a new kind of collective intelligence.
As we navigate this transformative era, the question now isn’t really whether to embrace this change, but how to steer it toward outcomes that enhance human capability, drive innovation, and ultimately create value.
Want to learn more about the power of AI agents for data analytics? Read more here about leveraging semantic layers for AI agents.
Get release updates delivered straight to your inbox.
No spam—we hate it as much as you do!
Related blog posts
Tool and strategies modern teams need to help their companies grow.

Novo Nordisk’s Transformation from 2D → 3D Commercial Operations AI Analytics
Key learnings from Novo Nordisk to help you along your journey to unlocking business value with AI in the commercial pharmaceutical space.

6 Gator-Sized Takeaways from the Gartner Data & Analytics Summit 2025
TLDR: agents on the rise, conversational everything, governance & data quality = king, driving/demonstrating ROI, the power of people never gets old.

Accelerating Data-Driven Decisions for All: Why Tellius Raised our $16M Series B
We just raised our $16M Series B funding round at Tellius ($33M to date) and couldn't be more excited! Read how we got here and what's next.